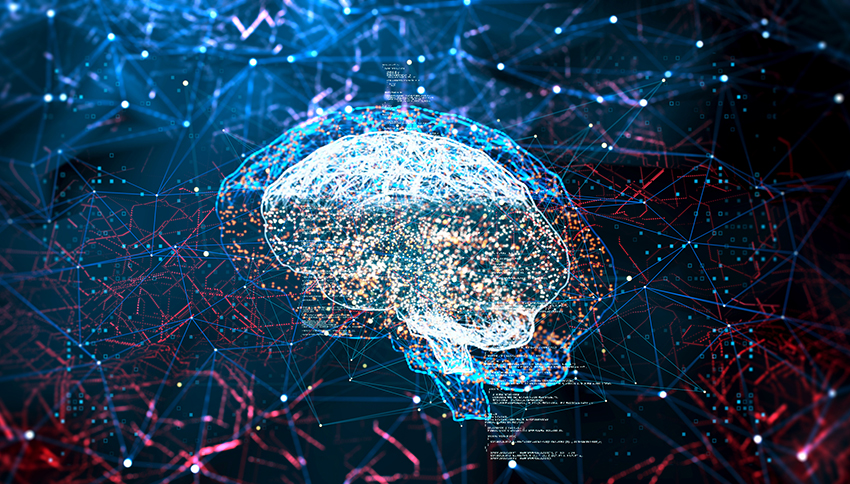
In 2019 alone, more than 1.3 million new citations were added to the 30 million existing abstracts and articles catalogued by PubMed, the NIH’s database of biomedical and life sciences journals and literature.
Each new entry, for the most part, contributes to the sum total of knowledge produced and validated by the world’s life sciences community.
Every entry, however, also serves as a reminder of how much remains to be understood about the astonishingly complex science of biology—from the intricate networks of biomolecules and molecular machines that underlie all of life’s processes to how their myriad interactions shape the behaviors of everything from cells and tissues to organisms and ecosystems.
Reverse engineering these processes gives scientists the best chance to understand human health and intervene in disease, but the human brain simply cannot keep up with this overwhelming volume of information.
In this era of big data, it is no wonder then that machine learning and other artificial intelligence (AI) methods, with a beyond-human ability to identify the subtlest patterns and connections in data at scale, have become essential tools in the quest to untangle the Gordian knot that is biology.
But what about the next era? For a group of researchers at Harvard Medical School’s Laboratory of Systems Pharmacology (LSP), a multi-disciplinary, cross-institutional effort to reinvent the science underlying the development of new medicines, the future utility of AI may not be as just a tool.
Instead, they are working to enable meaningful collaboration between humans and machines—using an AI system that reads essentially everything in PubMed and automates scientific discovery.
Developed by a team led by Benjamin Gyori and John Bachman, both research associates in therapeutic science at the LSP, and Peter Sorger, the Otto Krayer Professor of Systems Pharmacology at HMS and director of the LSP, the system text-mines enormous volumes of scientific literature. It then extracts information about causal mechanisms, creates models and generates predictions about biological interactions that human scientists can go on to test.
Earlier this fall, Gyori received a young faculty award from the U.S. Defense Advanced Research Projects Agency (DARPA) to advance their ambitious efforts. Moving toward what the agency dubs the third wave of AI, Gyori and colleagues aim for their AI method to soon be capable of learning and creating explanations based on contextual reasoning—similar to how human brains work.
Harvard Medicine News spoke with Gyori about his vision for the future of AI in scientific research.
Q&A with Benjamin Gyori
HM News: Could you describe what you and your colleagues working on?
Gyori: Nowadays, everybody is faced with a flood of information. It’s impossible to process it all, but we still have to somehow make rational decisions.
It’s the same for scientists. Something like 4,000 new publications appear on PubMed every day, and we have to figure out what to do next. Machines and AI in general can help us make sense of this flood of data.
The main aim of my project is to build a machine that monitors scientific literature and extracts new findings that could meaningfully change our way of thinking about a specific research question. We can use this knowledge to come up with new ideas, hypotheses and experiments.
I'm also specifically interested in human-machine collaboration—systems and interfaces that allow a human and a machine to have a conversation about a research problem.
HM News: What would be the impact of this kind of AI on scientific research?
Gyori: We think it’d be kind of an ultimate research assistant.
It would help us understand specific problems in biology and ask new questions in a way that is informed by and grounded in the large and sometimes intractable underlying scientific literature.
We can envision, for example, a quick human-machine dialogue with an AI model embedded with comprehensive scientific and patient data on COVID-19 to generate a hypothesis for a drug candidate that we could test in the lab.
A machine partner could provide key ideas to help scientists interpret results and design their next experiment in a rational way. In some scenarios, it could help clinical decision-making by revealing how a complicated cell signaling pathway interacts and connects to patient data.
I think it could even help resolve some of the important issues in reproducibility and controversy in science by monitoring and measuring the effect of new discoveries on our collective body of knowledge.
HM News: This sounds super futuristic. Can something like this really be achieved?
.jpg)
Gyori: We’ve actually already built the underlying machinery, called INDRA (Integrated Network and Dynamical Reasoning Assembler). It’s a pipeline that takes text from scientific papers and abstracts and creates computational model representations.
We use natural language processing systems, built by collaborators who specialize in text-mining, to read sentences and extract causal mechanisms—for example, which molecules activate what other molecules in a given signaling pathway.
But scientific findings come from many different sources, so this creates a large bag of disconnected facts that can often be contradictory or overlapping and have missing information or random errors.
The INDRA system takes these fragments of information and aligns them in a rational way to identify distinct pieces of evidence that point to the same underlying mechanism. It is also able to recognize generalizations of the same facts.
After a lot of error correction processes, it produces a knowledge base of causal mechanisms that resolves most issues of overlap, redundancy and contradiction. It also grades the various pieces of information it extracts and estimates whether something is a reading error or a high-confidence finding that’s likely to be correct.
Lastly, it turns this knowledge into something that a human can use, like models of networks of biochemical interactions that allow you to find mechanistic paths between a drug and a readout, for instance.
HM News: What exactly is this system reading?
Gyori: We built infrastructure to collect all the scientific literature that is published every day, run the reading systems and store the extractions at scale—tens of millions of publications in total, with thousands of new papers appearing on PubMed every day. We’ve exacted somewhere around 10 million unique mechanisms from these sources.
We’re focused on scientific publications right now. This includes PubMed abstracts and full texts when available, some licensed content and preprints to the extent possible. In principle, this framework would be easy to extend to other things like Wikipedia, we just haven't implemented it yet.
HM News: Will it be important to read other types of literature?
Gyori: Yes, this is one of the key new ideas in my recent DARPA award. I believe that in order for a machine to truly be able to use models for reasoning about biology and science, it needs to be able to understand the scientific context in which models operate.
Most models of biological systems or other complex systems focus very much on causal mechanisms that link things to each other—primarily the interactions between a set of specific proteins or molecules.
But we don’t necessarily connect these models to the broader scientific knowledge surrounding them—for instance, the mutation rate of a gene in a disease or data from clinical trials that are attempting a combination of drugs that target one of the proteins.
There's a world of scientific information that are not causal but that surround a model.
HM News: What does it mean for a machine to understand scientific context?
Gyori: This is one of the key gaps between a human scientist and a machine. The machine can happily simulate a system of differential equations representing the evolution of 1,000 biochemical species, but it has a gap in understanding the actual scientific context of what these species represent. This context doesn’t necessarily come from the scientific literature directly but rather from a wide range of databases and datasets.
There are many examples, like the Cancer Genome Atlas, which is an enormous collection of data on dozens of different cancer types that’s essentially a comprehensive atlas of cancer genomic profiles. There’s DrugBank for detailed data on thousands of different approved and experimental drugs and drug targets. Another good example is ChEMBL, which has bioactivity data on more than a million compounds.
If an AI system can connect these kinds of data with causal information that it exacts from the literature, it can embed its models with a much broader scientific context.
HM News: This still seems like an incredible amount of information for a human to handle. How would human-machine collaboration work?
Gyori: To interact with this much knowledge, you can’t just open it up and browse it. But there are already many applications and websites out there that let you interact with large biomedical resources. You go to a website, enter some parameters in a search form and get some results back. This is how scientists currently access information, whether it’s on PubMed, cBioPortal for Cancer Genomics, or whatever else.
What this lacks is the ability to follow up, to take an answer and follow it up with next steps. This is really where human-machine dialogue can help.
Essentially, we’re developing systems on top of INDRA so that users can ask the machine a question, get a result and ask follow-up questions referring to previous results. In this way, you can sequentially interrogate the underlying machine knowledge and models.
I think this back-and-forth, human-machine interaction is a much more productive and effective way to explore that information.
Ben Gyori demonstrates how a human-machine dialogue based on the INDRA system would work.