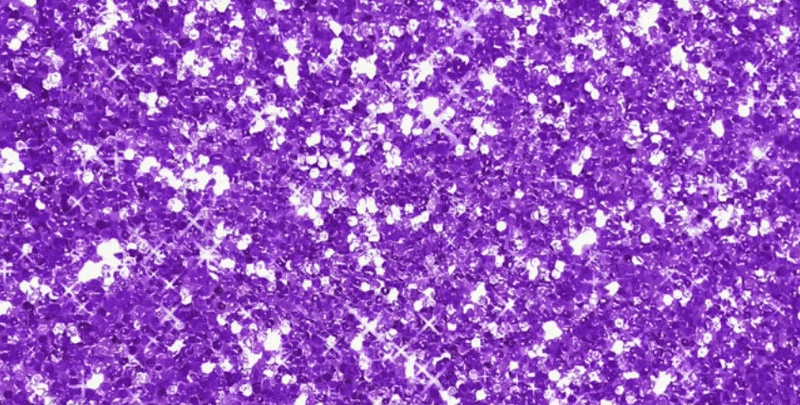
At a glance:
The new approach marks a major step forward in the design of AI tools to support clinical decisions in cancer diagnosis, therapy.
The model uses features of a tumor’s microenvironment to forecast how a patient might respond to therapy and to help inform individualized treatments.
The model can expedite the identification of patients not likely to benefit from standard treatments used in some forms of cancer.
Scientists at Harvard Medical School have designed a versatile, ChatGPT-like AI model capable of performing an array of diagnostic tasks across multiple forms of cancers.
The new AI system, described Sept. 4 in Nature, goes a step beyond many current AI approaches to cancer diagnosis, the researchers said.
Current AI systems are typically trained to perform specific tasks — such as detecting cancer presence or predicting a tumor’s genetic profile — and they tend to work only in a handful of cancer types. By contrast, the new model can perform a wide array of tasks and was tested on 19 cancer types, giving it a flexibility similar to that of large language models such as ChatGPT.
While other foundation AI models for medical diagnosis based on pathology images have emerged recently, this is believed to be the first to predict patient outcomes and validate them across several international patient groups.
“Our ambition was to create a nimble, versatile ChatGPT-like AI platform that can perform a broad range of cancer evaluation tasks,” said study senior author Kun-Hsing Yu, assistant professor of biomedical informatics in the Blavatnik Institute at Harvard Medical School. “Our model turned out to be very useful across multiple tasks related to cancer detection, prognosis, and treatment response across multiple cancers.”
Authorship, funding, disclosures
Co-authors included Xiyue Wang, Junhan Zhao, Eliana Marostica, Wei Yuan, Jietian Jin, Jiayu Zhang, Ruijiang Li, Hongping Tang, Kanran Wang, Yu Li, Fang Wang, Yulong Peng, Junyou Zhu, Jing Zhang, Christopher R. Jackson, Jun Zhang, Deborah Dillon, Nancy U. Lin, Lynette Sholl, Thomas Denize, David Meredith, Keith L. Ligon, Sabina Signoretti, Shuji Ogino, Jeffrey A. Golden, MacLean P. Nasrallah, Xiao Han, and Sen Yang.
The work was in part supported by the National Institute of General Medical Sciences grant R35GM142879, the Department of Defense Peer Reviewed Cancer Research Program Career Development Award HT9425-23-1-0523, a Google Research Scholar Award, a Harvard Medical School Dean's Innovation Award, and a Blavatnik Center for Computational Biomedicine Award.
Yu is an inventor of U.S. patent 16/179,101 assigned to Harvard University and served as a consultant for Takeda, Curatio DL, and the Postgraduate Institute for Medicine. Jun Zhang and Han were employees of Tencent AI Lab.