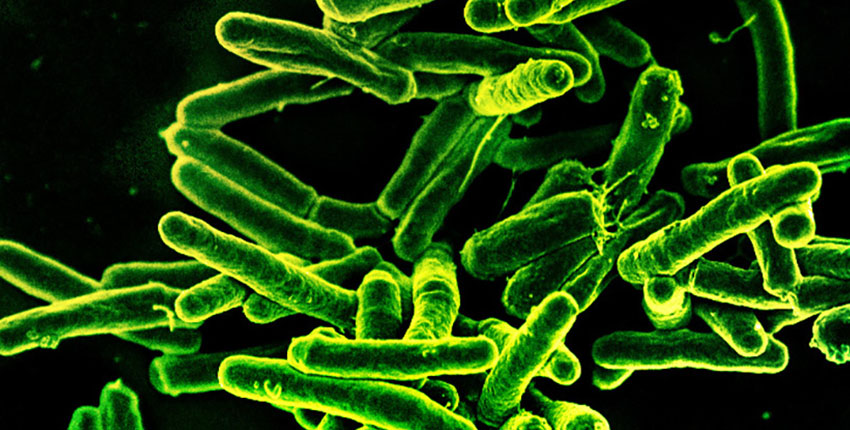
At a glance:
Highly localized TB strains are less infectious in cosmopolitan cities and more likely to infect people from the geographic area that is the strain’s natural habitat.
The findings offer new clues for tailoring preventive treatments after exposure to TB based on strain-host affinity.
The research provides the first controlled evidence that TB strains may evolve with their human hosts, adapting to be more infectious to specific populations.
For some forms of tuberculosis, the chances that an exposed person will get infected depend on whether the individual and the bacteria share a hometown, according to a new study comparing how different strains move through mixed populations in cosmopolitan cities.
Results of the research, led by Harvard Medical School scientists and published Aug. 1 in Nature Microbiology, provide the first hard evidence of long-standing observations that have led scientists to suspect that pathogen, place, and human host collide in a distinctive interplay that influences infection risk and fuels differences in susceptibility to infection.
The study strengthens the case for a long-standing hypothesis in the field that specific bacteria and their human hosts likely coevolved over hundreds or thousands of years, the researchers said.
The findings may also help inform new prevention and treatment approaches for tuberculosis, a wily pathogen that, each year, sickens more than 10 million people and causes more than a million deaths worldwide, according to the World Health Organization.
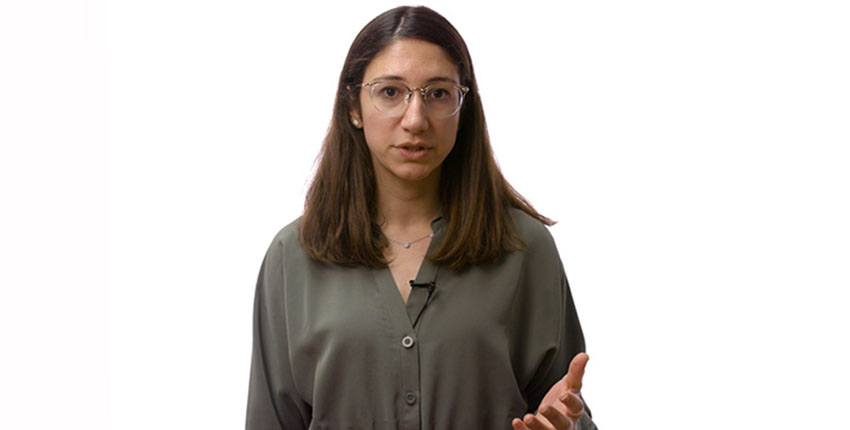
Authorship, funding, disclosures
Additional authors include Roger Vargas Jr of HMS; co-first author Francy Johanna Pérez-Llanos and Susanne Homolka of Research Center Borstel, Borstel, Germany; Roland Diel of University Medical Hospital Schleswig-Holstein; Vincent Escuyer and Kimberlee Musser of the Wadsworth Center, New York State Department of Health; Shama Ahuja, Lisa Trieu, Jeanne Sullivan Meissner, and Jillian Knorr of the New York City Department of Health and Mental Hygiene, Bureau of Tuberculosis Control; Dick van Soolingen and Don Klinkenberg of the National Tuberculosis Reference Laboratory, Center for Infectious Disease Control, National Institute for Public Health and the Environment (RIVM), Bilthoven, The Netherlands; Peter Kouw of the Department of Infectious Diseases, Public Health Service, Amsterdam, The Netherlands; Wojciech Samek of the Department of Electrical Engineering and Computer Science, Technical University Berlin and the Department of Artificial Intelligence, Fraunhofer Heinrich Hertz Institute, Berlin, Germany; Barun Mathema of the Mailman School of Public Health, Columbia University; and Stefan Niemann of the German Center for Infection Research, Partner Site Hamburg-Lübeck-Borstel-Riems, Borstel, Germany.
This work was funded by the U.S. National Institutes of Health/National Institute of Allergy and Infectious Diseases (grant R21AI154089), the German Research Foundation (GR5643/1-1), the BIH Charité Junior Digital Clinician Scientist Program funded by the Charité – Universitätsmedizin Berlin, the Berlin Institute of Health at Charité (BIH), the Leibniz Science Campus EvoLUNG (grant W47/2019), the German Research Foundation under Germany’s Excellence Strategy – EXC 2167 Precision Medicine in Inflammation, and the German Ministry of Education and Research (BMBF) for the German Center of Infection Research (DZIF).