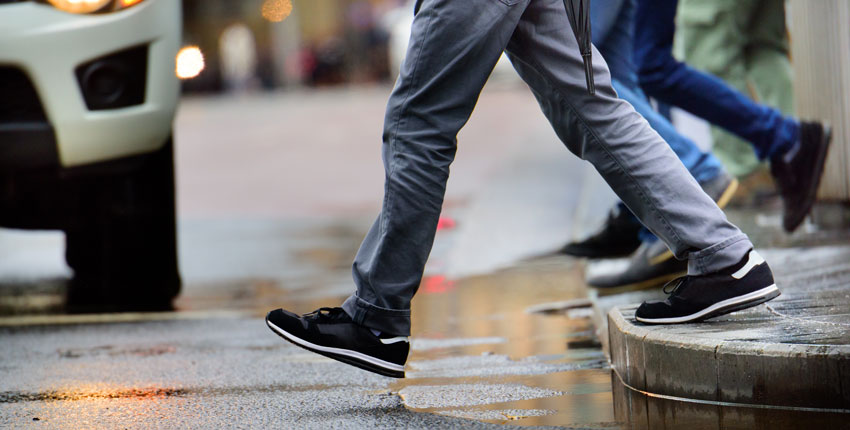
Our brains help us make countless decisions every day, from choosing whether to cross the road to selecting the most efficient route to the supermarket. Yet many of these decisions, even those that require our brains to factor in multiple sources of information at the same time, happen so quickly that we’re barely aware of the process involved.
Jan Drugowitsch, assistant professor of neurobiology in the Blavatnik Institute at Harvard Medical School, is intrigued by this process. As a neurobiologist with a doctorate in machine learning, he uses a computational lens to study how the brain operates.
He is particularly interested in how the brain takes in information about the world and uses this information to inform behavior. Drugowitsch’s lab focuses on theory, teaming up with experimentalists to test theories using computational tools.
In a conversation with Harvard Medicine News, Drugowitsch delves into the details of his research on how the brain processes information to make split-second decisions. He also discusses the role of computation and the importance of collaboration in unraveling the mysteries of decision-making.
HMNews: What aspects of the brain and behavior are you studying?
Drugowitsch: A lot of our work focuses on sensory perceptions on very short timescales—from milliseconds to seconds—and how we turn those perceptions into decisions. For example, an everyday human experience is making a decision about crossing the road. To do this, we need to figure out if the traffic situation is safe, including whether we have enough time to cross before a car arrives.
For most people, this decision happens in an unconscious way using different sources of information, such as the traffic flow on the left and right and the sound of oncoming cars. In my lab we are studying processes like this one that happen automatically and efficiently in the brain. We’re asking, how does the brain combine multiple sources of information across time to make these kinds of decisions?
Over the last few years, we’ve been studying increasingly complex domains of how we make these choices. We’ve shown that many of these choices follow principles of statistical decision-making because the information we have is uncertain, so we have to gauge different sources of information against each other and ask, “Are we certain enough to commit to a choice?” My lab has been formulating statistical models that capture the process, including complexities such as the trade-off between speed and accuracy.
Now, we are shifting to understanding more continuous behaviors such as navigation. For example, keeping track of direction during navigation is a process that doesn’t have discrete steps—we keep track of our direction on a constant basis, and use this information to make behavioral decisions. We want to know how the brain does this on a continuous timescale.
HMNews: You use computational tools in your research. What is computational neuroscience?
Drugowitsch: There are currently two forms of computational neuroscience. Traditional computational neuroscience involves building models in the language of mathematics, physics, and engineering to describe hypotheses about how the brain performs computations. These computations are usually related to how the brain processes information about the world.
There is also a newer form of computational neuroscience that has emerged with the ability to gather much larger datasets about the brain. This kind of computational neuroscience involves developing and using more sophisticated tools to process complex neural data. We use both in our work.
A focus of my lab is how humans and animals deal with uncertain information. Essentially all of the information that we have about the world is uncertain, and handling uncertain information moves us into the realm of statistics. We use a lot of tools from statistics because they provide the adequate language to talk about beliefs about things in the world. More specifically, we use Bayesian statistics to formulate models of how uncertain information is processed in the abstract sense. Then we use tools from physics to define how this information processing that we’ve worked with on a statistical level can be realized in the brain. This is where biology comes in—it introduces constraints on how the brain operates and how it executes these statistical computations.
HMNews: Your recently published paper in Neuron about navigation in the brain uses some of the above approaches. Can you tell us a bit more about this work?
Drugowitsch: Our research builds on an earlier experimental observation about place cells, a population of cells in the hippocampus of the brain that represent our location in space. This observation, made in mice and rats, is that while a rodent is standing still, place cells suddenly become active in a rapid sequence of bursts that seems to simulate the animal’s trajectory through the environment. There are two hypotheses about the role of this activity. One is that it helps us memorize what we’ve done before and move it to long-term memory. The other is that it helps us plan future navigation.
Before addressing these hypotheses, we wanted to refine our understanding of what these bursts actually do by understanding the data better. We used existing data on rats foraging for food in a two-meter by two-meter environment and applied Bayesian statistical methods to gain a fuller picture of activity in place cells.
Previously, scientists thought that only a small subset of the bursts in place cells stimulated trajectories through open environments. However, we found that the majority of bursts are part of these trajectories. Additionally, the trajectories of these bursts feature momentum as if the animal were actually moving through space, even though it’s stationary.
This is interesting because earlier work on activity of place cells during sleep found that the trajectories of those bursts don’t feature momentum. Thus, our findings suggest that bursts of activity in place cells may play a fundamentally different role depending on whether an animal is awake or asleep. Now that we have this information, we can move back to building computational models to understand how place cells help us plan and navigate through the world.
HMNews: Why do you think neuroscience is moving in a computational direction?
Drugowitsch: I think the adoption of more computational tools is in part a response to the many possibilities nowadays for collecting complex data. Previously, if we recorded from a single neuron while an animal did a simple task, we could interpret our data without using complex models. Now, we routinely record from hundreds or thousands of neurons in the brain while animals perform complex tasks, leading to data that can only be analyzed with complex computational models.
There has been a realization that most neuroscientists need at least a basic understanding of how these computational models work, which has created a push towards greater literacy in computational neuroscience.
To this end, I co-direct a certificate program in computational neuroscience for graduate students at HMS. The program started because we noticed an increasing demand for students to learn quantitative skills, yet the courses we offered in this area weren’t broad enough.
Our aim is to develop new courses that provide students with the skills they need to understand the full array of computational tools being developed to analyze neuroscience data. We also want to increase cohesion of the computational neuroscience community at HMS, and provide more forums where students can discuss questions in the field.
HMNews: What motivated you to pursue computational neuroscience?
Drugowitsch: I wanted to become a computational neuroscientist because I strongly believe that understanding the brain requires a complexity of thinking that cannot be achieved by intuition alone—and a lot of traditional experiments rely on intuition.
Very often I find that things are different than I expected, which strengthens my belief that we should build formal models of how the brain operates in order to make progress in our understanding. Formulating these models expands our ability to think about complex interactions in the brain that are beyond what we can hold in our heads. We’re outsourcing this complexity to tools that have been developed in math and physics.
In general, I’m driven by curiosity, trying to figure out new things and trying to discover the principles that define how we operate.
In my lab, we like to ask specific questions because this is the only way to make experimentally testable predictions. However, we hope to discover general principles that underlie these questions. If we are studying how an animal performs particular behaviors, we try to extract a generalization from that specific situation that we can test in another set of experiments. Computational neuroscience gives us the tools we need to explore these questions.
HMNews: In your work, you often team up with colleagues from other branches of neurobiology. Why?
Drugowitsch: Building theories and running experiments require a different set of skills, so collaborations allow theorists like me to work with gifted experimentalists in a fruitful way.
There are many theories in computational neuroscience that remain untested, so by collaborating with experimentalists we can test those theories to see if they are supported by the data.
In some cases, we work with scientists running experiments with humans. The benefit of human experiments is that the training is fast—humans can perform complex tasks right away. The disadvantage is that it’s hard to look into their brains.
For other questions, especially those about specific neural connections, we collaborate with scientists studying animals. For example, we’re working with Rachel Wilson, who studies drosophila [fruit fly] neurophysiology. We are asking, how does a specific neural circuit in the drosophila brain perform specific computations? We hope that the motifs we discover can be generalized across species, including humans.
In my lab, we may be able to develop blue-sky theories, but at the end of the day we need to connect those theories to data gathered in the real world. Working with people who conduct experiments allows us to do that.
This interview was edited for length and clarity.